Topic 16 K-Means Clustering
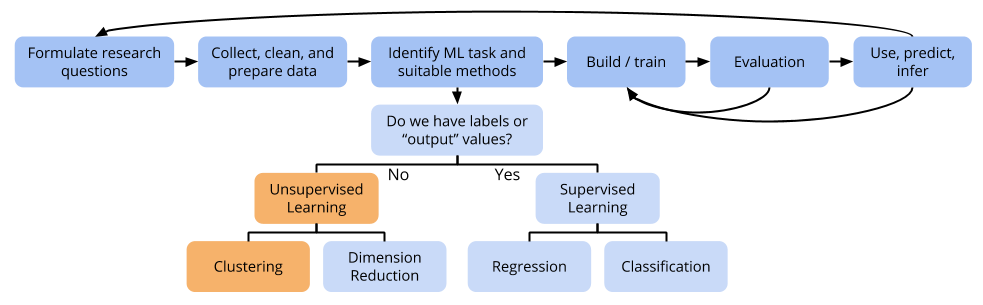
16.1 Discussion
Applications of clustering
- Biology: Are there species, subspecies of these organisms based on their gene expression, physical measurements?
- Chemistry: Are there different groups of compounds? Perhaps based on shape, toxicity to humans?
- Sports: Are there different type of players? Based on skill, play style, body type?
- Market analysis and economics: Are there certain type of consumers? Purchasing behaviors?
- Geography: Are there certain types of neighborhoods?
Clustering seems like classification, but is different
- In classification, the response variables are used in training the model. The response variables supervise how the model is fit.
- In clustering, there might not be a response variable, and we don’t need to use it even if there is. e.g. In the
iris
dataset, there is a column indicating the flower species, but nothing dictates that the species level (as opposed to subspecies) should be our scientific interest.
Considerations in picking the number of clusters/evaluating clusters
- Context!
- Also plots of number of clusters versus total within-cluster sums of squares
- The combination of the two helps inform the number of groupings that are reasonable for the observed data
- Evaluating clustering is hard!
- It’s a joke (but kind of true) that with enough tweaking of clustering parameters, distance metrics, scaling…you could get any clustering that you desired.
- We need to look at multiple variations of the clustering: different methods, parameters.
- What makes sense? e.g. Both 3 clusters and 4 clusters might make sense on different levels. Perhaps you discover that 3 clusters roughly corresponds to level of binge-purchasing behavior and that 4 clusters roughly corresponds to purchasing aligning with 4 distinct categories of hobbies.
Prediction with clustering
- Once you have a set of clusters, it’s possible to assign new cases to the closest cluster.
- You might find after enough new cases that new clusters might be needed. Re-cluster the data. How do things change? What new insights are gained?
Clustering predictors as opposed to cases
- When we cluster cases, we look at distances between cases (distances between rows of our dataset).
- When we cluster predictors, we look at distances between predictors (distances between columns of our dataset).
- So clustering predictors involves transposing our dataset.
- PCA is another way to “cluster” predictors. Coming up next!
16.2 Exercises
You can download a template RMarkdown file to start from here.
NOTE: completing these exercises is a part of your Homework 7, due Wednesday, April 10 at midnight.
To iron out the K-means algorithm, we’ll look at a small example of physical measurements (sepal length and width) of 5 irises in the first exercise:
irises <- data.frame(
Width = c(0.5,0.5,2.3,2.8,3.0),
Length = c(2.0,2.5,1.3,1.8,1.3)
)
ggplot(irises, aes(x = Width, y = Length)) +
geom_point() +
geom_text(aes(label = c(1:5)), vjust = 1.5) +
lims(x = c(0,4), y = c(0,4))
Cluster these 5 flowers by hand using K=2 clusters. For the first stage of initialization, use the random cluster assignments shown below in the
cluster
column. Show your work for 2 iterations of the centroiding and reassignment steps.cbind(irises, data.frame(cluster = c(2,1,1,1,2)))
## Width Length cluster ## 1 0.5 2.0 2 ## 2 0.5 2.5 1 ## 3 2.3 1.3 1 ## 4 2.8 1.8 1 ## 5 3.0 1.3 2
You may find it helpful to use this function to calculate the distance of each case to a specified point:
dist_iris <- function(point) { sqrt((irises$Width - point[1])^2 + (irises$Length - point[2])^2) } # For example: distance of every case to (1,2) dist_iris(point = c(1,2))
The
kmeans()
function in R performs K-means clustering. Check your work from the previous exercise.irises_clust2 <- kmeans(irises, centers = 2) # Check out the cluster assignments irises_clust2$cluster # Plot the clusters irises <- irises %>% mutate(cluster = irises_clust2$cluster) ggplot(irises, aes(y = Length, x = Width, color = as.factor(cluster))) + geom_point() + lims(x = c(0,4), y = c(0,4))
In the remainder of the exercises, we’ll look at the full iris
dataset of 150 flowers.
data(iris)
- Part of performing K-means clustering is choosing an appropriate value for K.
To explore the impact of K, perform a K-means cluster analysis of the
iris
data using justSepal.Length
andSepal.Width
(for ease of visualization). Use the following code for \(K=2\) and adapt it to explore \(K=3\) and \(K=20\).# Take only Sepal.Length & Sepal.Width iris_sub <- iris %>% dplyr::select(Sepal.Length, Sepal.Width) # K=2 clusters km_clust2 <- kmeans(iris_sub, centers = 2) ggplot(iris_sub, aes(x = Sepal.Width, y = Sepal.Length, color = as.factor(km_clust2$cluster))) + geom_point()
Based on these plots, discuss the pros and cons of low and high K.
- Before moving on, let’s get more comfortable working with unfamiliar objects in R.
- Look at the help page for the
kmeans()
function and scroll down to the “Value:” section. What is the name of the component of akmeans
object that contains a measure of total within-cluster variation? - Whenever we want to extract a certain component of an R object that is a list, we can use the code
list_object$name_of_component
. Display the measure of total within-cluster variation forkm_clust2
.
- Look at the help page for the
- A strategy for picking K is to compare the total squared distance of each case from its assigned centroid (the
total within-cluster sum of squares
) for different values of K.Write a for-loop to calculate the total sum of squared distances for each K in \(\{1, 2, ..., 50\}\). Plot these squared distances versus K.
# Create storage vector for total within-cluster sum of squares tot_wc_ss <- rep(0, 50) # Loop for (i in 1:50) { # Perform k means clustering on iris_sub ??? # Store the total within-cluster sum of squares tot_wc_ss[i] <- ???$??? } plot(1:50, tot_wc_ss)
Based on this plot, which values of K seem reasonable? Explain.
Extra! Think about the scientific questions that you want to answer for your final project. Could clustering play a role in your scientific pursuits?